目的
データのグラフ化について、どのツールを用いれば迷うことが多いので、
それぞれのグラフの基本的な書き方をまとめていこうと思います。
今回は代表的な下記の2つについて基本的な内容について記載します。
- Matplotlib
- Seaborn
グラフ化するデータ
下記のデータを選択し、右クリック→コピーする
Date | JPN_SPY | JPN_GLD | JPN_TLT |
2021/3/1 | 4128943 | 5169606 | 4502003 |
2021/3/2 | 4099413 | 5201180 | 4505277 |
2021/3/3 | 4051520 | 5152047 | 4463696 |
2021/3/4 | 4026074 | 5132857 | 4462526 |
2021/3/5 | 4125351 | 5167594 | 4498671 |
クリップボードに保存したデータを取り込む
df = pd.read_clipboard()
df = df.set_index('Date')
Matplotlib
概要
Matplotlibはかなり低レベルのツールで、データの表示、凡例、タイトル、目盛りのラベル、注釈といった基本コンポーネントからプロットを組み立てられています。
メリット
最も基本的なツールなので、ネット上にも多くの記事が存在しており、参考にしやすい。
デメリット
設定事項が多いため、コードが多くなってしまう。
グラフの見栄えがあまりよくない。
公式サイト
インストールするもの
pip install pandas
pip install matplotlib
pip install seaborn
モジュール読み込み &グラフ表示
import matplotlib.pyplot as plt
plt.plot(df)
実行結果
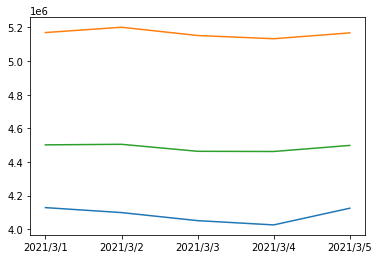
グラフ設定表示
#グラフを作成する準備。空のプロットウインドの作成
fig = plt.figure()
plt.plot(df)
#グラフ設定
#全体のタイトル
fig.suptitle("Date & Value")
#ラベル
plt.xlabel('Date')
plt.ylabel('value,yen')
実行結果
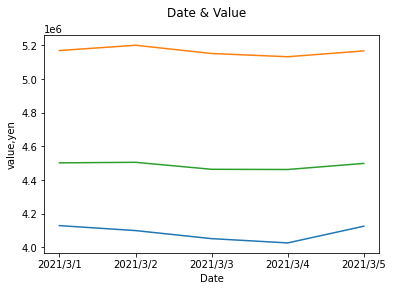
複数グラフ作成
複数グラフフォーマットの作成
#グラフを作成する準備。空のプロットウインドの作成
fig = plt.figure()
#2×2グラフのフォーマットを作成。
#fig.add_subplot(〇行, 〇列,〇番目)
ax1 = fig.add_subplot(2,2,1)
ax2 = fig.add_subplot(2,2,2)
ax3 = fig.add_subplot(2,2,3)
ax4 = fig.add_subplot(2,2,4)
plt.plot(df)
#グラフ設定
#全体のタイトル
fig.suptitle("Date & Value")
#ラベル
plt.xlabel('Date')
plt.ylabel('value,yen')
4つ作成したグラフフォーマットのうちの最後のもの(4番目)にグラフが描画されます。
同様にグラフの設定も最後のグラフにしか反映されません。
実行結果
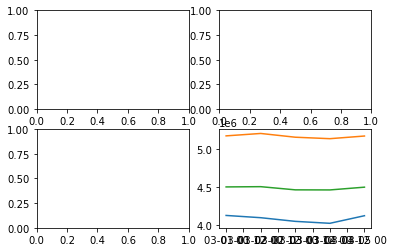
空欄の枠へのグラフプロット
fig = plt.figure()
ax1 = fig.add_subplot(2,2,1)
ax2 = fig.add_subplot(2,2,2)
ax3 = fig.add_subplot(2,2,3)
ax4 = fig.add_subplot(2,2,4)
#空欄の枠に各グラフをプロット
ax1.plot(df['JPN_SPY'],color = "blue")
ax2.plot(df['JPN_GLD'],color = "orange")
ax3.plot(df['JPN_TLT'],color = "green")
plt.plot(df)
#グラフ設定
#全体のタイトル
fig.suptitle("Date & Value")
#ラベル設定
plt.xlabel('Date')
plt.ylabel('value,yen')
#文字を斜めに表示
plt.xticks(rotation=45)
ここで空欄の枠に各グラフをプロットします。
ただ、x軸ラベルへの設定は、このままでは4番目のグラフにしか反映されません。
実行結果
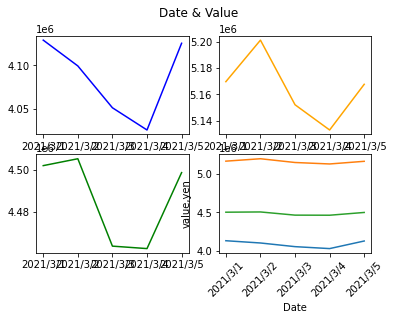
複数グラフへの表示設定
fig = plt.figure(figsize=(12,6))
ax1 = fig.add_subplot(2,2,1)
ax2 = fig.add_subplot(2,2,2)
ax3 = fig.add_subplot(2,2,3)
ax4 = fig.add_subplot(2,2,4)
#空欄の枠に各グラフをプロット
ax1.plot(df['JPN_SPY'],color = "blue")
ax2.plot(df['JPN_GLD'],color = "orange")
ax3.plot(df['JPN_TLT'],color = "green")
plt.plot(df)
#グラフ設定
#全体のタイトル
fig.suptitle("Date & Value")
#複数グラフへの設定
for i in range(4):
plt.subplot(2, 2, i+1)
#ラベル設定
plt.xlabel('Date')
plt.ylabel('value,yen')
#文字を斜めに表示
plt.xticks(rotation=45)
#グラフの重なりを修正
plt.tight_layout()
for文により複数グラフに設定を反映します。また、グラフのサイズおよび重なりを修正します。
細かい設定など多まだまだありますが、いったん完成とします。
実行結果
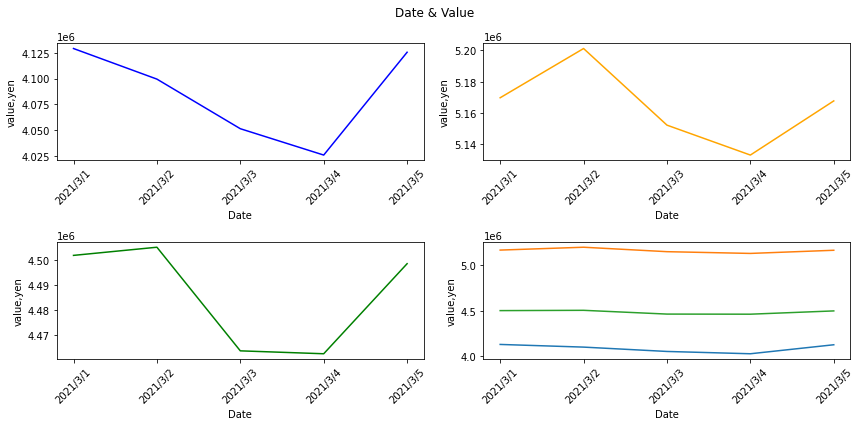
Seaborn
概要
Seabornは、Matplotlibの上位互換のようなものでプロットがより見やすく、より美しくなります。
メリット
短いコードで見やすいグラフが書ける。
デメリット
Matplotlib と比較すると参考記事が少ない。
インポートするもの
pip install pandas
pip install matplotlib
pip install seaborn
Matplotlibモジュール読み込み &グラフ表示
import seaborn as sns
sns.lineplot(data = df)
1行でそれなりのグラフを書くことが可能です。
実行結果
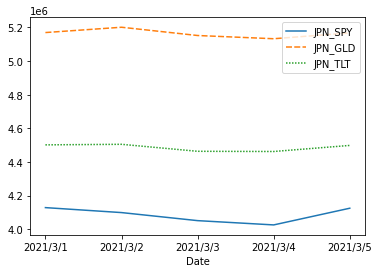
複数グラフ準備作成
fig = plt.figure()
ax1 = fig.add_subplot(2,2,1)
ax2 = fig.add_subplot(2,2,2)
ax3 = fig.add_subplot(2,2,3)
ax4 = fig.add_subplot(2,2,4)
sns.lineplot(data=df['JPN_SPY'],color = "blue", ax =ax1)
sns.lineplot(data=df['JPN_GLD'],color = "orange", ax =ax2)
sns.lineplot(data=df['JPN_TLT'],color = "green", ax =ax3)
sns.lineplot(data=df, ax =ax4)
実行結果
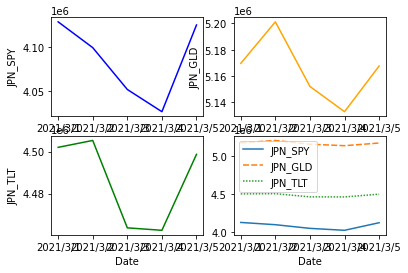
グラフ表示設定
fig = plt.figure(figsize=(12,6))
ax1 = fig.add_subplot(2,2,1)
ax2 = fig.add_subplot(2,2,2)
ax3 = fig.add_subplot(2,2,3)
ax4 = fig.add_subplot(2,2,4)
sns.lineplot(data=df['JPN_SPY'],color = "blue", ax =ax1)
sns.lineplot(data=df['JPN_GLD'],color = "orange", ax =ax2)
sns.lineplot(data=df['JPN_TLT'],color = "green", ax =ax3)
sns.lineplot(data=df, ax =ax4)
#グラフ設定
#全体のタイトル
fig.suptitle("Date & Value")
#ラベル設定
plt.ylabel('value,yen')
#凡例の位置調整
plt.legend(bbox_to_anchor=(1.0, 1.0), loc='upper left')
#グラフの重なりを修正
plt.tight_layout()
実行結果
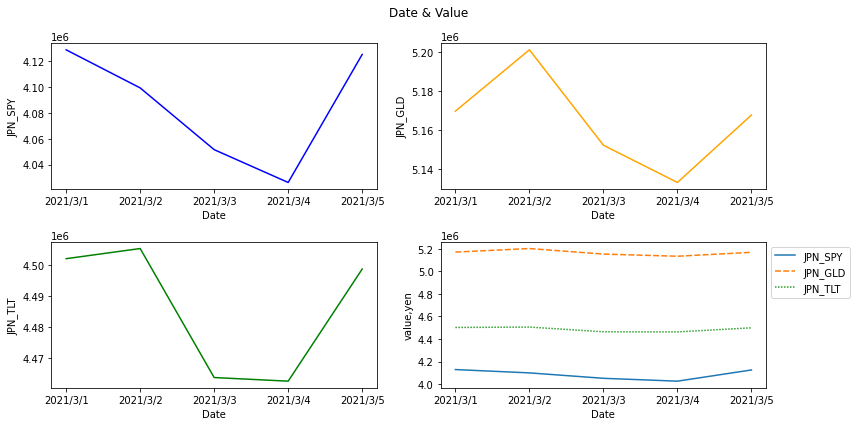
Seabornの方が短いコードで、見た目の良いグラフをかけることが可能なことがわかります。